- Automation IT
Summary
Fast Forward
- A large amount of equipment can be simultaneously supervised with only a few sensors.
- Cloud-based machine learning algorithms can detect upcoming failures.
- An acoustic monitoring system prevents any interference with the safety and control system of the plant.
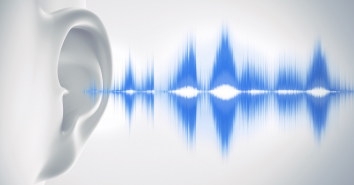
Deployed using a safe and secure cloud approach
The monitoring systems used today in hydropower plants are based on a number of sensors that are directly mounted to the machines. The monitoring systems generate alarms if certain threshold values are exceeded. These thresholds are normally static limits based on technical standards or based on experience during the commissioning phase.
An acoustic monitoring system enables continuous monitoring of a large number of components and equipment simultaneously with only a few sensors. Applying cloud-based machine learning algorithms enables the detection of anomalies and clustering of typical machine behaviors.
The acoustic monitoring system emphasizes security and privacy regarding communication and prevents any interference with the safety and control system of the hydropower plant by design, as only one-way data transfer away from the hydropower plant is enabled. It further provides a cost-effective solution for monitoring existing hydropower plants, because it requires no physical modifications of the equipment itself.
Challenges of hydropower plants
One major challenge is the remote location of many hydropower plants. Some are very hard to reach, while others are not reachable in wintertime or during bad weather conditions like a monsoon.
Another challenge relates to the level of expertise and staff training in rural areas. Most big hydropower development projects therefore require extensive training of local labor for the safe operation of the plant.
Furthermore, the continuous reduction of maintenance personnel in recent years and decades affects plants even in well-structured areas. Many plants are unmanned and are only visited for inspection purposes in intervals of weeks or months. This raises the concern that something could happen the day after an inspection round has been finished, and then go unnoticed and cause severe damage over time.
Smart monitoring systems, such as the proposed acoustic monitoring system, will autonomously monitor the running equipment. A requirement of such systems is the data connectivity of these power plants, which is traditionally a point of concern. Connections routed through the Internet, in particular, are regulated by national standards and laws. However, as a customer pointed out, "solutions that address this concern are technically possible but still face a psychological hurdle."
Different monitoring approaches
Classical monitoring
Typical hydropower plant monitoring systems are fully integrated in the control system of the plant. Each monitoring sensor focuses on a singular quantity at a specific location. The sensors are physically mounted to the equipment. Therefore, a retrofit requires some physical modifications of the equipment.
During the design phase of the monitoring system, the engineer predefines static alarm limits, for example, in accordance with a norm like DIN ISO 7919-5, where maximum relative vibration displacement limits are defined. These limits are derived from statistical analysis of many hydropower plants. Although they gather the collective hydro experience, the limits are not necessarily suitable for a particular plant or even power unit. Adjustments to these static limits are usually done during the commissioning of the hydropower plant.
Advanced integral monitoring
Often, before equipment failure, early indicators like noise, heat, or odor are noticeable, even before a classical monitoring system observes an indication in a specific signal, because the static alarm limit is not yet reached. Equipment failure starts very early, progresses slowly, and is only noticeable to either personnel inspecting the plant or where slowly changing trends are monitored explicitly. This also requires that the actual failing component is equipped with a sensor. Often, this is not the case, because too many sensors would be required.
The new approach is based on microphones, which collect integral information from a larger space or a larger set of equipment, like inside the turbine pit. The system can detect anomalies or deviations from typical machine behavior and slowly moving trends and can diagnose specific signal patterns and relate them to past events. Figure 1 shows a sketch of the system where sensor data is combined with process information (e.g., current active power). Such an approach becomes feasible today, because the storage concepts and algorithms required to implement them are now available.
Some of the intelligence of this monitoring system is based on domain-specific preknowledge, like the knowledge of typical machine frequencies and their meaning. But, to achieve diagnostic or even predictive capabilities beyond such preknowledge, there are three major steps that have to be taken during the so-called learning phase.
- First, collect a large number of training samples to get a fingerprint of the "normal" situation. This is the basis for detecting any abnormal situation or anomaly.
- Second, classify samples that show anomalies by the type. Later, if a reasonable number of such anomalies have been found, then the classification of similar types can be done automatically.
- Third, do further classification, where the technical background of a certain sound event is included. This puts the sample in a specific technical context. This technical classification includes the analysis of actual events happening locally as well as past experiences of either the involved experts or those already stored in the system.
Only now can the system be trained to diagnose specific events in addition to detecting anomalies. Prediction is still one step further. This requires that the classification explains whether the specific sound is an indication of an actual event to happen in the future.
First applications of advanced integral monitoring approach
Audible noise–An early indicator of failures
Audible noise is an early indicator of mechanical problems in equipment. A typical mechanical system starts rattling or making other noises before it breaks. Hence, microphones were selected as a first integral measurement quantity to develop the new type of monitoring system. Audio detection has been installed in pilot sites for a long-term test under real conditions.
Start-up sequence analysis
To give an idea of what can be heard and diagnosed, the diagram shows a start-up sequence of a hydropower unit that was equipped with five measurement units in different locations across the hydropower unit (figure 3). Assuming the start-up sequence of the plant is fixed, the algorithm can now match any future start-up sequence and immediately issue a warning if it is not in accordance with the reference case. It is also noticeable that some of the events do not influence all signals in the same way. This is due to the spatial separation of the measurement locations.
Thus, the collected information only becomes meaningful and useful for diagnostics with the actual technical context of the installation. Interpretation of acoustic data relies not only on machine learning but also on a large amount of domain knowledge.
Potential failures to be captured
The table has examples of events that were indicated by audible noise upfront (figure 4). The collection is based on experience over the years and a selection of recent cases. Some of the cases actually led to an unplanned stop of the machine with major repair work required. It is interesting to note that the examples are not limited to a specific type or size of machine. In a number of cases, an early indication by acoustic monitoring would have prevented major damage and significantly saved maintenance costs.
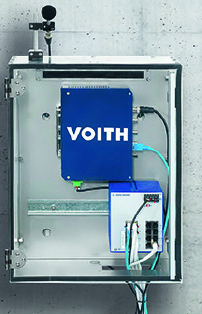
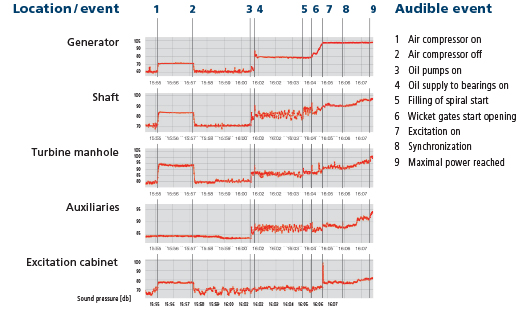
Safety, security, and privacy for cloud-based approaches
Cloud-based software, network, and computing technologies bring opportunities to gain knowledge from vast amounts of data, but also bear certain risks. The safety of a hydropower plant must not be compromised. It is ensured by the following:
- The integral monitoring system performs only non-mission-critical operations.
- Only one-way data transfer away from the hydropower plant is enabled.
- The monitoring system uses communication channels that are separate from standard process control.
These measures make sure that the integral monitoring system does not interfere with any plant safety and protection, control, or supervisory control and data acquisition (SCADA) systems. Depending on the user's requirements and trust in information technology (IT), these principles can be implemented either as software or hardware. Still, communication across the Internet is needed to establish a dataflow between the cloud computing system and the monitoring system on site.
Connection of advanced monitoring systems to the cloud
One critical part of information security is the router, which separates the monitoring network from other networks in the direction to the Internet. The VPN router is set up so that it cannot be connected from "outside." It will simply refuse all connection requests. Separate, dedicated communication channels are strictly built up by the monitoring system and the router themselves. They contact the cloud actively and via tunnels, not the other way around. Being not actively reachable from "outside" is an important measure to increase security.
Each OnCare.Acoustic device on the user side, for example, is equipped with a private certificate. Thus, the cloud can identify the connecting devices, as it holds their public key. Additional security is provided by the fact that cloud components also have a private certificate, so devices can verify their intended connection counterpart.
Routers with integrated firewall and certificates are important cornerstones of security during data transport. However, they are supported more by measures, like encryption, which are standard in modern communication, such as AMQP, which was first used in the banking and finance sector.
Connecting advanced monitoring systems to the power plant
Another critical part is the connection between the OnCare.Acoustic system and the control system or SCADA system of the user. The system provides better diagnostics if it knows about the operating conditions of the power units. Thus, a data interface is required to retrieve process data from the SCADA or control system. Here, we have multiple possibilities. And this is also where the North American Electric Reliability Corporation (NERC) Critical Infrastructure Protection (CIP) standards for cybersecurity have to be consulted.
The cybersecurity standards of NERC are aimed at protecting "critical infrastructure" by law. They are enforced in the U.S. for large power plants, for example. But their existence also increases the awareness of IT security topics in smaller plants and influences other industries.
Depending on the total network layout of the plant, measures can be used to permit data transfer only in one direction: from SCADA or the control system to OnCare.Acoustic. Such measures may include:
- Routers for network separation and with communication traffic control
- Protocol converters to connect to existing historians or other data layers on the customer side, instead of connecting directly to the SCADA or control system
- Data diodes, and especially physical data diodes. In this case, the connection is established through a single fiber optical channel. Thus, sending data toward the SCADA or control system is physically impossible.
Therefore, part of the scope of such new monitoring systems is a thorough site assessment to determine the best possibilities for IT security.
Promising results
The proposed solution shows promising results and offers opportunities to improve the availability of hydropower plants by reducing the risk of unplanned outages. It allows plants to recognize degrading equipment early and supports maintenance crews by guiding them to the proper area inside the hydropower plant. It thereby has the potential to also reduce operational risk and maintenance costs.
By combining the solution with remote expert services, it can also continuously guide local crews in gaining experience and expert knowledge faster. It is easy to retrofit for existing hydropower plants, even without existing monitoring systems. Unmanned power stations and hydropower plants in remote areas with only limited accessibility especially benefit from such an advanced and integral monitoring approach. It is a safe and secure solution due to the network separation from any existing SCADA or control system.
Building on the experience gathered from the first long-term installation of the system, similar, if not the same, algorithms can be applied to existing data from classical monitoring systems. This will have even greater potential to detect possible equipment failures. Overall, enriching the existing portfolio of monitoring solutions by cloud-based approaches brings new benefits to the operators of hydropower equipment and will lead to predictive maintenance strategies in the future.
Reader Feedback
We want to hear from you! Please send us your comments and questions about this topic to InTechmagazine@isa.org.