- By Ian McGregor
- July 31, 2020
- Discrete Mfg & Machine Control
Summary
- User-specific simulation models enhance operational understanding and guide solution choices.
- Fully tested systems can be delivered on time and on budget
with offline digital twin controls verification. - Virtual reality remote solution demonstrations and operator training are both safer and cheaper than face-to-face meetings.
Virtual analysis of dynamic digital twins reduces automation system design risks
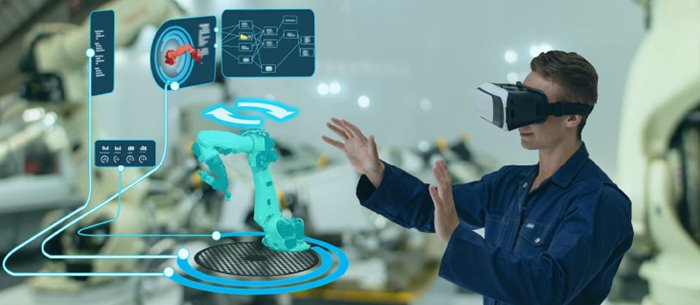
By Ian McGregor
Industrial simulation and emulation are powerful but still underemployed techniques for designing, developing, and testing better automation solutions and machines. When used as a central part of a project’s workflow, they can significantly shorten the project development and commissioning time, reduce overall costs, and provide numerical justification for design decisions. The ongoing emergence of many Industrial Internet of Things (IIoT) technologies has enhanced their appeal and effectiveness by creating much excitement about the opportunities offered by digital twins, but also generated some degree of confusion around their application and areas of impact.
Challenge of making the right design choices
We take it for granted today that systems used in manufacturing and distribution must be automated for them to be competitive. Automation is how we eliminate manufacturing errors, reduce delivery times, keep retail outlets stocked, and ensure that orders placed on a website arrive at their destination just a few hours later. The physical side of automation is familiar to us—conveyors, autonomous vehicles, robots, and so on. Even outside of industry, these items are recognized and are often employed as icons of contemporary life.
This visible face of automation masks the complexity of the system, however. It is the advances made in control systems that have enabled the growth in automation we benefit from today. Until recently, advances were evolutionary rather than revolutionary, but now we are faced with a range of opportunities promising a step change made possible by the concurrent emergence of several complementary technologies. IIoT brings together practically unlimited computing power, cheaper sensors and data storage, big data, and better standards, among other things. But how does an automation system design team choose from the wide range of material handling solutions, control, and management systems available, and how do they demonstrate they have made the right decisions?
Using dynamic digital twins to develop better solutions, faster
Work done on improving operational technology through the intelligent use of real-time data to create digital twins currently gets much industry attention, as does the ability to diagnose and maintain operating machines using remotely supported technicians equipped with augmented reality systems. However, current technology also offers many important opportunities in the design, analysis, and commissioning phases of an automation project, prior to ramp up and operation. Like quality, the best automation solutions are designed in from the start. The correct use of technology not only leads to robust and flexible systems, but also stays within budget. Engineers use dynamic models to create virtual representations of proposed systems in order to understand, improve, and demonstrate future operations in a repeatable manner.
To create the big picture, first answer all the small questions
The creation of a fully operating system model is a demanding discipline in itself, as it requires answers to all the operational questions before the model can be completed and run. As a robust design process, the creation of a dynamic digital twin is second to none and results in an intuitive, interactive, and understandable representation of the physical and logical objective. The model represents the current state of the project, accessible to all team members, and becomes the trusted reference. Suggested changes should be tested against the core model in order to understand any related consequences and the overall impact the changes might have on operation and throughput. By providing repeatable and robust statistical results, these models help project stakeholders reduce the risk associated with their investment, and therefore increase the likelihood of the implementation of further successful automation.
How simulation shortens the design cycle and leads to better outcomes
The engineering starting point for any automation system is an operational specification of some sort, including a definition of required throughput. The path from there to the finished system is a series of decisions, each of which must have a justification and set of reasons behind it. The decisions may be about material handling technology choices, or storage options, or how best to batch and route similar orders, but each outcome has consequences and costs associated with it. For simpler decisions, logic or a spreadsheet calculation is often all that is required. But many industrial systems are highly complex, incorporating many concurrently changing and interconnected subsystems that do not lend themselves conveniently to spreadsheet analysis or easy explanation, and this is where dynamic simulation is invaluable.
Discrete event simulation is a powerful tool for industrial analyses of this type—it facilitates the breaking down of a large problem into a data-driven model of many smaller systems, each of which is able to be tested and verified in a repeatable way. Models are event driven, in the same way that much of the real world operates—when orders arrive at an e-commerce center, for example, they are entered into the control system, which initiates a cascade of actions that eventually lead to the orders being delivered.
The model focus is invariably on product or load flow and its consequences—throughput, storage, queues, resource use, and so on. The actions taken follow the business rules of the facility, which must be accurately represented in the model. Models are used to test different ways of dealing with orders to determine which provides the best outcome. Even complex models run considerably faster than they would in real time, and experimental runs can be distributed across many computers or run in parallel in the cloud to get useful results faster.
Demonstrate, experiment, understand, improve
Simulation models of this type serve several purposes. Initially, they are an accurate functional representation of each part that makes up the complete system. Models contains cycle times and decisional logic in order to demonstrate the operation, and as they run, they help stakeholders understand the parts and the whole, often accelerating development.
They also serve as an impartial judge between experience and opinion. Through guided experimentation they help to eliminate fruitless discussion about which is the best of several options by the generation of repeatable results. Their purpose is to develop and dimension the “best” solution to reach agreement on layout, capacity, and so on. From this point, validated models are a means of understanding the system response under any number of data sets representing peak throughput, error conditions, or efficient normal operation, for example. Not only are they used for testing product mix and resource allocation, but the study of their operation can lead to definitions of best practice, degraded performance under various conditions, and recovery from shutdowns.
Test performance of higher-level control software against the simulation model
Simulation models are data-driven, dynamic digital twins of the mechanical and logical system being developed, and they are a reliable way to understand how the various elements constituting the real system will interact. Models can be connected to higher-level order management, manufacturing, or stock controllers to test their performance against the digital twin under a range of foreseeable operating conditions and increasing confidence in the technology application.
Value of simulation for flexible automated system design
The benefits of using simulation to create a flexible dynamic digital twin are many and varied—ranging from material handling equipment selection, queue dimensioning, resource allocation, and operational decisions to recovery procedures and system management selection. In short, any throughput test that would be useful to carry out on the real system can be carried out at lower cost and without disruption or danger on the dynamic digital twin.
Simulation and emulation differences, and why it matters
Simulation models are mathematical representations of complex industrial systems designed to help understand and improve the real thing. They can be connected to external data sources, such as order management or stock control systems. So why complicate things by introducing the word “emulation” (rather than “simulation”) when the system is connected to an external logic controller?
First and foremost, simulation and emulation models have different objectives, and this fundamental difference brings with it implications for the whole structure of the model. Simulation models are used to experiment with different scenarios, often involving changes to many physical and logical parameters such as layout, business rules, and order profiles. Simulation models need to execute quickly if they are to be useful, and they take a “load-centric” view of the world, where each load follows decisional logic to traverse the system. The load is the active element that drives the simulation model forward.
Whilst this is appropriate for the understanding of product flows and resource use in a complex system, it is not how automation systems work. Emulation models are used to test and debug high- and low-level control systems, with the aim of taking the task off the project’s critical path and carrying it out virtually, and in parallel with the system build. To do this, emulation models must be able to connect to control systems and respond to them as they run in the same way real equipment does. Emulation model elements need to be functionally close to their real counterparts, including sensors and motors—they are true digital twins in that respect.
Loads move in an emulation model as a result of their presence being detected by sensors and the control system executing logic, which is designed to operate equipment and machines to process or move them. Whereas in a simulation model the logic and business rules are approximated and completely contained within the model, an emulation model is connected to and driven by the real control system. As a result, emulation models run in real time to ensure accurate responses. Even in the rare case of a control system not containing timers, it is still unadvisable to run the model faster than real time, as this may create unrealistic responses.
While simulation models are load-centric, emulation models are equipment-centric. The focus of an emulation model is much more precisely defined, and the movement of modeled loads is the consequence of virtual equipment being activated or deactivated, in a close parallel to the real world.
How does the IIoT benefit simulation and emulation?
Industrial model building requires data, and the better the data, the better the outcome of the model. The IIoT has prompted an awakening to the value of an accurate digital representation of physical assets, as well as enabling access to better and more cost-effectively stored data collected by cheaper sensors. This serves the requirements of both simulation and emulation. Simulation models are massively data driven, which applies at several levels:
- Initial model build, where the physical layout plays a central role in product movement and therefore throughput, and where each model element may require not only product or action-specific cycle time data but also changeover times
- Resource availability, such as shift and break schedules, breakdown rates, repair times, and maintenance patterns
- Order schedules, pick lists, manufacturing, or assembly schedules.
The IIoT offers opportunities to aggregate real data anonymously, whether for specific machines or generic processes, and this is central to building more accurate models. Simulation experiments can generate a large quantity of data that is traditionally hard to absorb and comprehend; being able to extract correlations and deduce causation is something that big data analysis techniques are very good at, and can do quickly. The results of multiple simulation runs can be stored and analyzed further using machine learning techniques to identify situations that could have been better resolved by modifying the control system in some way.
Real opportunities offered by virtual and augmented reality
The augmented or virtual reality headset (often referred to as XR for convenience) has become a familiar symbol for all things concerning the digital thread and IIoT, and while it is not as central to the successful application of this new technology as its prominence in related publications suggests, it certainly is a useful tool in many circumstances. The first of these is clearly the communication of complex ideas, as demonstrated by a running model. It is valid to ask how a model viewed using an XR headset is better or more effective than watching the same model on a monitor. The answer is perhaps self-explanatory to anyone who has had first-hand experience of it, but for those who have not, it can be summed up as immediacy. Assuming that the person in the headset is not among the small percentage of users who feel nauseous under those circumstances, their first experience is generally one of agreeable surprise, if not astonishment. The environment is unreal yet convincing, and the feeling of “being there” is compelling, informative, and useful.
Multiple users can experience the same model simultaneously and see and communicate with each other within it. They can be in different offices, states, or countries, and meet virtually within it to inspect the current state of development and then decide next steps. A mixture of green screen and XR enables real operators to demonstrate the complete operation of virtual prototype semiautomatic machines, with viewers able to check cycle times and even change position to verify clearances whilst discussing the task with the operator.
This approach reduces development time and costs—no more need to find a mutually convenient day to take a flight and stay a night in a hotel just to sign off on the next phase after a meeting, which could have been held virtually and sooner, and maybe lasted only twenty minutes.
XR offline training – Safer and more comprehensive
No XR headset is complete without controllers, which allow users to navigate around inside the model, operate controls through browser-based human-machine interfaces (HMIs) and control panels, and interact with products. At this point, the model becomes a functional training tool that is safer and less disruptive to ongoing production than the real thing. Recovery procedures from malfunctions, which may be costly or even dangerous to operators and equipment in the real world, can be carried out without consequence in the virtual world.
Achieve better automation systems using simulation modeling and emulation
Making simulation and emulation a central part of the design and testing workflow introduces a productive discipline to the creation of automation systems. This framework requires all relevant elements and ensures they work together—highlighting weaknesses yet to be resolved and focusing stakeholders on the current state of the project. From initial ideas to a fully investigated solution, simulation accelerates the process and makes sure the chosen result is robust and verifiable. Controls testing using an emulation model enables increased control over the project timeline and a more thoroughly tested system, delivered on time. Beyond the design and development phase, both models remain valuable for operator training and to evaluate and develop any future modifications.
As the project manager of a large pharmaceutical company put it, “An emulation model is the first place the two truths of an automation system meet—the mechanical truth is the CAD, and the logical truth is in the form of the control system.” By bringing them together to test in a virtual environment early in the design cycle, you can eliminate the need for later alterations in the real world and be ready for ramp-up and production.
The near-term future will unite IIoT and existing product architectures more closely to the benefit of users; the direction of development is dictated by the dual goal of a more user-specific experience and a more fully featured and robust framework to facilitate this. The objective for all is a fuller deployment of client-matched solutions that help generate cost-effective and robust automation solutions, on time and on budget.
Reader Feedback
We want to hear from you! Please send us your comments and questions about this topic to InTechmagazine@isa.org.