- By Lisa Graham
- Process Automation
Summary
Fast Forward
- Reasons to implement PAT include reduced cost, improved reliability, and better quality.
- Leading challenges are changing company culture and accessing and analyzing data.
- Two case studies show the results of applying advanced analytics to PAT projects.
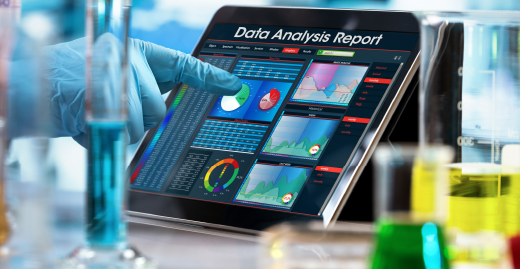
Operationalizing advanced analytics for data-driven decision making
Leveraging process analytical technology (PAT) advancements helps companies derive value by combining data from process and analytical instruments with advanced analytics to:
- empower subject-matter experts (SMEs)
- augment process development and scale up
- optimize the globally connected system
- realize the potential of the Industrial Internet of Things (IIoT/Pharma 4.0)
- reduce manufacturing cycle time
The concept of PAT has grown into a broad field encompassing process analysis, chemical engineering, chemometrics, modeling, process automation and control, and knowledge and risk management. This approach is consistent with the FDA's document titled Guidance for Industry PAT, which is "written for a broad industry audience in different organizational units and scientific disciplines" and "discusses principles with the goal of highlighting opportunities and developing regulatory processes that encourage innovation."
From an innovation perspective, a strong PAT methodology includes a plan for connection to disparate data sets, advanced analytics, and the culture change required to actively implement insights through improved workflows. This is important, because PAT should ultimately support data-driven decision making, which requires a firm grasp of measurements, data science, and analytics workflows-along with a plan for summarizing and disseminating the knowledge gained (figure 1). Further, the value of the outcome should demonstrate a return on investment whether the analyses are using an effective PAT methodology for diagnostic, predictive, monitoring, prescriptive, or descriptive views (figure 2).
In this article, case studies will illustrate an effective use of a PAT methodology for optimizing the facility operations and active pharmaceutical manufacturing processes required to produce quality medicines for patients. But first, let's look at why PAT is important for improving pharmaceutical manufacturing processes.
Background: Why PAT?
The demand for new therapies, loss of revenue from biosimilar and generic competition, and the rapid growth of emerging markets are forcing manufacturers to be more productive. To meet these challenges, pharmaceutical companies must capture process parameters and real-time measurements of critical quality attributes using analytical techniques and data analysis/modeling techniques, often referred to as PAT. A robust PAT methodology is essential to achieve:
- cost effectiveness:
- increase system automation and control
- reduce or eliminate production of waste
- enable the use of alternative or less expensive raw materials
- reduce production cycle times
- reduce energy use
- support a semi- to fully continuous processing approach
- reliability:
- achieve target quality consistently
- prevent rejection of batches
- reduce time from manufacture to product release
- demonstrate confidence in product quality
- productive system performance:
- simplify and shorten development cycles through increased process understanding
- enable extended automation capability
- achieve greater throughput with minimal additional time or resources
- incorporate real-time release to reduce or eliminate many testing requirements
PAT can help achieve these goals through process analysis, including the application of field-deployable instrumentation and chemometrics to monitor chemical or physical attributes, and the detection of events that cannot be derived from conventional physical measurements like temperature or pressure. Process analytical instrumentation can be organized into many categories: (1) physical property analyzers measuring attributes like viscosity, refractive index, and thermal conductivity, (2) combustion analyzers, (3) electrochemical analyzers measuring voltage or current that correlates with concentration, and (4) spectrophotometers that measure an attribute via electromagnetic interactions.
Examples of using spectroscopic technologies for determining material characteristics in situ for traditional pharmaceutical processing include the determination of drug content uniformity during powder blending and tablet manufacture, and drug-layering during pellet coating. Active pharmaceutical ingredient manufacture in reactors and cell growth/protein expression in bioreactors use in situ methods like focused-beam reflectance and dielectric spectroscopy, respectively, to monitor product attributes in real time.
Another example of innovation in process analytical instrumentation and methodology is the recent implementation of a mass spectrometry-based approach to simultaneously monitor the extensive array of product quality attributes present with therapeutic molecules. This approach has successfully enabled the real-time monitoring of bioreactors and quality control release, and it has the potential to replace several conventional electrophoretic and chromatographic methods currently used to release therapeutic molecules. The development of this new method is a prime example of proactively reevaluating the desired data source, and then transitioning away from using earlier PAT measurements that are less directly connected to the protein attributes.
Deploying these and other types of process analytical instrumentation to gather data is the first step. The second is using advanced analytics software to derive insights and improve operations. This data analytics component of a strong PAT methodology consists of empirical, multivariate, and first-principles modeling techniques, including mechanistic modeling, chemometrics, and statistics packages.
Challenges: Data access, ease of analysis and company culture
Although not always accurate, historical data can often bring insight into the future performance of a process. In development, for example, identifying which unit operations are robust and which are not, along with the effect on quality metrics, is key for defining the required work to shorten the scale-up process while ensuring a quality-by-design approach ready for filing.
For example, the relationships of the process inputs to the respective critical quality attributes must be determined to define the design space of a process. General challenges in using PAT effectively include:
- Access to all the relevant data: Data connectivity continues to be a tremendous source of frustration for getting the most value out of PAT. For example, an important aspect of PAT is its role in supporting an effective quality-by-design approach for therapeutic molecule manufacturing, which requires a deep, molecular-level understanding of the attributes crucial to the safety and efficacy of the medicine. Quite often, these datasets are either maintained off-line or trapped in a process data historian. In either case, it takes extra effort, often in the form of spreadsheet gymnastics, to bring the datasets together and make them available to the engineer or scientist performing the data analysis. Applications that connect to and present data from a multitude of disparate data sources, including process historians, should be a core element of the PAT implementation strategy.
- Ease of data analytics and visualization: The ability to view data from both the process instrumentation and the process analytical instrumentation together in one place during select times of operation is crucial for performing investigations and monitoring, and for developing accurate models. Analytics techniques, including multivariate- and first-principles-based modeling, only work well when the PAT methodology provides:
- a centralized, single location to overlay multiple experiments or multiple conditions from within an experiment
- automated file transfer from off-line instruments to eliminate cutting and pasting various data files into spreadsheets
- handling samples from different time intervals, including analysis when a sample may have been missed or skipped
- easy batch definition with the ability to search by key metadata about the experiments
- automated templates to apply standard data views and calculations for quick routine analysis, tied in with report generation
Figure 3 illustrates how engineers can selectively query data to identify stages of operation. For example, when an analytical instrument is being tested or recalibrated, the data signal no longer represents the actual process. To develop a robust model, the engineer should be able to exclude these periods of bad signals from the analysis. Further, the best data analytics application can support the engineer in developing a model to predict what the values should have been if the sensor had been operating properly.
- Ensuring a dynamic culture that is ready to embrace change: Realizing the full potential of the PAT methodology often requires a fundamental change in company mindset from the top down and the bottom up. This is especially true in groups that have not already implemented agile data collection and an analysis methodology in their manufacturing process. In these cases, the group needs to review workflows to understand the existing obstacles for collecting and accessing the right data, and then use the right data analytic software. This software must be able to perform the required analysis and be implemented so subject-matter experts can use it, as opposed to only data scientists. A successful PAT methodology is evidenced by a system that the full organization can use (figure 4), but this often requires the adoption of new workflows.
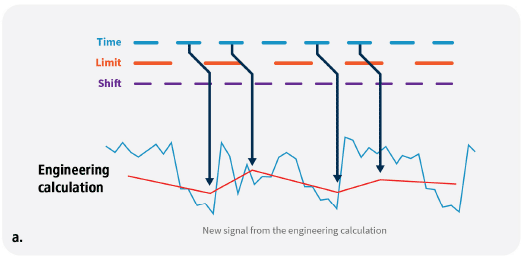
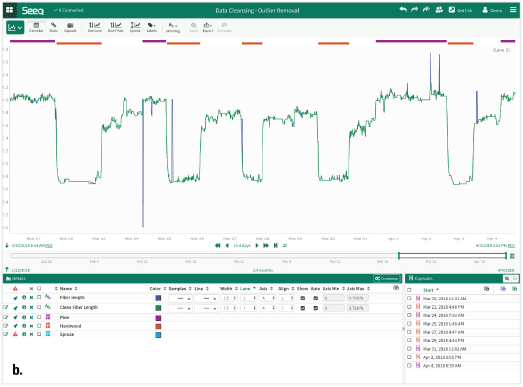
Advanced analytics in action
For robust, quality products, facilities operations and product manufacturing processes must both run smoothly. On the facilities operations side, examples of unit operations include water purification, filtration, heating, and cooling. On the product manufacturing side, examples of pharmaceutical unit operations include:
- crystallization in reactors and protein expression in bioreactors
- filtration and purification (e.g., chromatography)
- lyophilization
- feeding and blending
- granulation
- tableting
- tablet coating
Both areas are subject to the inherent challenge of enabling engineers to apply their domain expertise to optimize the operation of equipment such as pumps, valves, compressors, and heat exchangers.
The following case studies illustrate the effective implementation of a complete PAT methodology. These case studies showcase both the facility operations and active pharmaceutical manufacturing processes required to produce quality products. For each case study, the PAT methodology being followed employs the following aspects:
- Proactive data type selection: Installing instrumentation to deliver the required data
- Connecting disparate data sources: Encompassing all relevant information, including process operations data and process analytical instrument data
- Investigation: Having the full range of data signals available for each process, batch, or asset readily available to:
- cleanse data
- add and remove datasets of interest
- define periods of interest using capsule logic
- perform profile searches to teach and find similar performance
- Advanced analytics to analyze and develop models: Using built-in and user-friendly tools for initial calculations and immediate application to new batches
- Construction of a full process train view: Comparing multiple different unit operations side by side to understand the effect of upstream changes on downstream operations
- Workflow documentation: Using journaling, annotation, and lab notebook functionalities to capture critical steps in the workflow
- Knowledge management: Communicating key results in a report containing a dashboard of current or previous batches or runs.
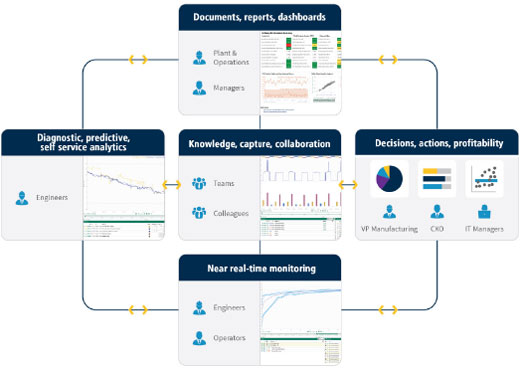
Case study A: Robust facilities operation
When operating a pharmaceutical-grade water system, it is imperative to have a direct line of sight to the current data and the historic trends. This requires continuous availability of process operations data and real-time particle and biologic counts. Benefits of using a well-defined PAT methodology in this case study include:
- enhanced quality assurance
- improved risk management
- energy savings
- reduced resource and labor requirements
- extended facility and equipment life
In this example, an online water bioburden analyzer was the primary process analytical instrument. This online and real-time technology simultaneously detected particles and determined biologic counts without requiring staining or reagents, or export of the data for upload into a process data historian.
With connection to all relevant data established, the organization performed analysis using advanced analytics software to quickly identify time periods outside sanitization cycles and tank filling cycles to assess the impact on bioburden and particle counts (figure 5). From these observations, statistical models were built, and 3 Sigma boundaries created to represent a robust operating space. To share the results, the organization documented workflows in Seeq Journal and created a dashboard in an Organizer Topic.
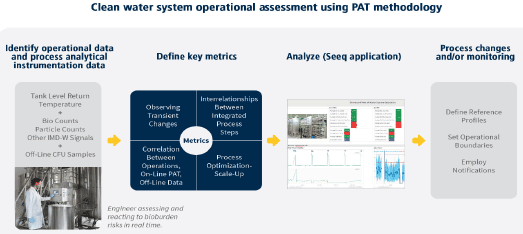
Case study B: Column maintenance in production-scale chromatography
A PAT methodology can be applied in production-scale chromatography to track column efficiency degradation using, for example, transition analysis and the calculated height equivalent to the theoretical plate (HETP), so engineers can optimize column maintenance. This example shows conductivity and volume signals spanning a variety of production, downtime, regeneration, and transition analysis periods (figure 6).
First, the organization uses Seeq's Profile Search tool to identify all of the transition analysis periods, enabling calculation of the HETP during each transition. The HETP increases, indicating fewer resolved separations per unit column length (i.e., lower efficiency), with total volume through the column, as might be expected. This calculation is refreshed as the connected data sources are updated, allowing engineers to continuously monitor column efficiency and schedule maintenance before the HETP exceeds the desired threshold, which would decrease product purity.
Similar analyses could be used to optimize flow rates and eluent composition, increasing productivity and reducing maintenance costs. Further, this information can be captured as part of a continuous improvement process through workflow documentation in Journals and Organizer Topics.
Recipe for success
In a world currently trapped in unfocused data collection and time-consuming, complicated, nontransferable spreadsheets, there is a path forward. Data in and of itself is not useful unless one has an approach to derive insights from it. Fortunately, there is a recipe for success.
The keys are implementing an advanced analytics solution where the datasets are gathered strategically with an end use in mind upfront, and then providing a way for these datasets to be easily accessed and analyzed within a user-friendly interface. With this approach in hand, organizations can more fully realize the knowledge management practices essential to achieving business value within a PAT framework.
Reader Feedback
We want to hear from you! Please send us your comments and questions about this topic to InTechmagazine@isa.org.