- By Russ Waddell
- Factory Automation
Summary
Fast Forward
- Manufacturing executives rank predictive analytics as the most important advanced manufacturing technology of the future.
- The decades-old vision of anticipating breakdowns before they happen can now be achieved.
- Factory connectivity coupled with the MTConnect standard is the underlying infrastructure of both predictive analytics and process improvement.
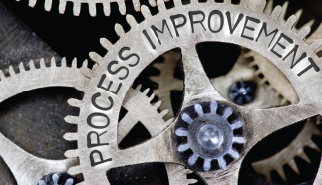
Process improvement, predictive analytics, and more
By Russ Waddell
In the Industrial Analytics 2016/2017 survey and report from the Digital Analytics Association Germany and IoT Analytics, machine predictive/prescriptive maintenance was the top industrial data analytics application. Similarly, in the 2016 Global Manufacturing Competitiveness Index by Deloitte and the U.S. Council on Competitiveness, top manufacturing executives ranked predictive analytics as the most important future advanced manufacturing technology.
With the MTConnect standard, manufacturing equipment provides data in structured XML, rather than in proprietary formats. Uniform data opens up a world of new applications for industry. MTConnect data sources include production equipment, sensor packages, and other hardware. Applications using MTConnect data have more efficient operations, improved production optimization, and increased productivity.
Within the MTConnect community, the rise of analytics has been watched closely. The MTConnect standard drastically reduces the cost of translating between different brands or types of equipment and devices. A factory outfitted with MTConnect is an appealing target for an analytics project, because data is already homogenized and uniformly defined. The MTConnect standard defines a semantic vocabulary or dictionary of terms, as well as a structure of how terms relate to one another. The dictionary specifies units, exact wordings and spellings, and definitions. For example, "spindle speed" is normalized to "RotaryVelocity" and expressed in units of revolutions per minute. The structure or data schema specifies that RotaryVelocity is nested under a set of one or more rotary axes (for a single- or multispindle machine), and that rotary axes are in turn nested under a set of all axes, including rotary and linear.
MTConnect for monitoring
Machine monitoring was the first widely commercialized application using MTConnect data, and the standard has become closely associated with shop floor monitoring software and the companies writing and selling it. Those applications have long been used to visualize data like utilization and overall equipment effectiveness, although these days most software packages have expanded to include additional data views, calculations, or functions, such as downtime classification or data breakdowns by shift, process, or operator. Many of these features relied on MTConnect data, either by adding calculation across multiple existing MTConnect data items or by adding new data items into the standard. Despite the close association between MTConnect and shop floor monitoring companies, MTConnect is not application specific. The role of the MTConnect standard is to expose device data using a consistent dictionary of terms (data items) and predefined structure, but once that data is formatted and exposed it could be used for anything.
Predictive analytics for the factory
Building on the foundation already in place for monitoring and status reporting, predictive analytics is the most talked-about upcoming application area for MTConnect. Predictive analytics, which broadly means using data and statistical modeling to anticipate future events or conditions, has been talked about for decades in manufacturing. It may not have always gone by the name predictive analytics, but forecasting and error prediction have long played a big role in mitigating production risks.
Predictive analytics in manufacturing starts with crosscutting predictive models that apply to many different industries. It is a discipline unto itself, and the best practices, modeling, software tools, techniques, and computer processing power serving the discipline are always improving. As data science has become a full-fledged industry and a lucrative career path, many in the field have set their sights on manufacturing. Meanwhile, data connectivity in factories has become far more common. Many companies with existing analytics capabilities in industries like finance, logistics, telecommunications, or ecommerce have been quietly seeking out new data sets to run through their algorithms and forecasting models. What starts as investigations into the Industrial Internet of Things (IIoT) often leads to industrial process improvement applications, which enjoy an appealing combination of high value-added use cases and available data. The result is a raft of new entrants into manufacturing from data analytics.
For established players in manufacturing and automation, the forecasting framework from finance, economics, and many other fields is already familiar. That framework has already been widely applied in measurement and test, quality and inspection, and statistical process control (SPC)\, among other manufacturing disciplines. As a result, many manufacturers are building up their analytics capability in house. Others are seeking close partners, especially from smaller companies with some manufacturing background, as well as programming and forecasting capabilities. Under the umbrella of predictive analytics, predictive maintenance is the most hotly pursued function. The concept is simple: the current maintenance paradigm is to fix or repair components once they have failed or based on a predetermined preventative maintenance schedule. In the future, predictive maintenance will dictate repair if and only if failure is about to occur.
Process analytics here today
Although predictive analytics is getting the most attention, a less glamorous area has the potential to be widely commercialized very soon. Analytics for process improvement is a logical next step from monitoring, and it avoids the complexity of building good predictive models. The 2016 IoT Analytics report uses the more precise but cumbersome category "analytics that support process automation," which was considered far less important than "predictive/prescriptive maintenance of machines" among survey respondents. Compared to predictive analytics, analytics for process improvements and automation also lacks some of the associated jargon. Machine learning, deep learning, neural networks, and artificial intelligence, for example, are critical leading-edge technologies for predictive models. Process analytics, though, could be as simple as process visibility helping a human operator, technician, or engineer remove tedious or repetitive manual steps. Process visibility is like shop floor monitoring in that it presents data that already existed but may have been hidden to operators, engineers, or management.
In custom automated systems, continuous manufacturing or packaging lines, and high-volume production work, it is already common to include current process data to users and management. This data may include detailed views of setups, operations, or individual parts and assemblies. Shop floor monitoring, by contrast, emphasizes a complete and comprehensive view of all equipment and assets. Companies are increasingly demanding both detailed process data and comprehensive asset management views.
In commercial software packages or those integrated into automated systems, process analytics manifest as feedback to operators or improved communication between production and engineering. For example, a technician may know to periodically remove a filter for visual inspection. The inspection interval may be set at the production station or dictated by engineering, but additional automated data collection and analysis supplements and improves the process. Switching from manual inspection logs to automatically recording filter removal events saves time, improves accuracy, and decreases the number of process steps for the technician.
Other application areas for process analytics include supplementing SPC and quality systems and tool path generation and tool selection for machining. In fact, there is barely a line between SPC and process analytics. Data collected for process analytics can simply be additional inputs to improving existing systems. For machining operations, linking data from a machine, cutting tool, CAM system, and even the part itself has huge implications for tooling. Established suppliers, new startups, researchers, and end users have all converged on better tool paths and better tool selection as near-term wins for analytics.
How analytics will come to market
In the near term, automated predictions will not replace human reasoning in the factory. Complex, nonlinear thinking gives humans the edge over algorithms for managing processes. Where predictive analytics shine today is in highly repetitive, high-volume operations where one or a few target variables with a big impact can be modeled. Temperature and vibration data collected from rotating parts, such as generators or turbines, is well suited for supplying data to predictive models. Those models will eventually adapt to handling more and more variation and work from smaller data sets. Human operators will still manage work with higher variation, but increasingly benefit from analytic insights. Computerized tips and inputs to streamlining processes are already available today; warnings about predictive maintenance concerns-with a human deciding when and how to act-is easy to picture in the not-too-distant future.
Manufacturers are more likely to develop process analytics applications in house than predictive analytics applications. Process data is often very manufacturing specific, whereas tools and techniques for predictive analytics are not necessarily dependent on close knowledge of processes. As a result, predictive analytics can be a back-office function or contracted out to software or platform vendors.
Standards landscape
Rapidly improving factory connectivity is the underlying infrastructure that allows both predictive analytics and process improvement. There is a huge demand for access to usable data, and a maturing data standards landscape in manufacturing that helps service that demand. More and more devices in the factory have a hardware or software plug for getting data out as well as just in. The data egress was initially very low-level signals, but software libraries and APIs help abstract from otherwise generic voltages, and semantic vocabularies like MTConnect standardize and supply additional context from one device to the next. The data models in MTConnect are most widely implemented in discrete manufacturing equipment, but have been used on everything from vending machines to discrete sensors to personnel data.
The standards ecosystem for manufacturing data extends well beyond MTConnect, which solves a relatively small and narrow problem for analytics. National manufacturing policies, including Platform Industrie 4.0 (Germany), Made in China 2025, and Make in India/Digital India, explicitly call out standards as enabling technology, in large part because clean and coherent data is required for the most promising next-generation applications. The broadest layer of the ecosystem is occupied by reference architectures like the Reference Architecture Model for Industrie 4.0 (RAMI) or the Industrial Internet Consortium's Industrial Internet Reference Architecture. These reference models specify functional areas that need industry (or cross-industry) collaboration and coordination to be successfully addressed.
In many cases, standards bodies are working to directly integrate standards. For MTConnect, this includes implementation guidelines for ISA-95/B2MML on device integration with higher level enterprise planning and management systems. It also includes the OPC UA/MTConnect companion specification, originally released in 2012 and currently being updated for a new version expected in the first half of 2018. The MTConnect development road map includes expanded device and asset models (e.g., robotics, additive, programmable grippers or work holding, and file transfer), but also covers integration with QIF quality standards and expanded functionality by supporting UPnP discovery.
Much more to do
Analytics is an area of tremendous opportunity for manufacturing and automation. Basic connectivity and data collection are increasingly the norm, and new applications that go well beyond status reporting are rapidly being commercialized. Meanwhile, the standards ecosystem for manufacturing data is evolving to serve demand for increasingly complex needs. Semantic definitions provided by MTConnect are part of the puzzle, but industrial policy in major manufacturing countries and global consortia are working to minimize duplicated effort. Everyone in manufacturing and automation should know that analytics are coming to the industry in a big way. Now it is time to get educated on how the hype is fast becoming reality.
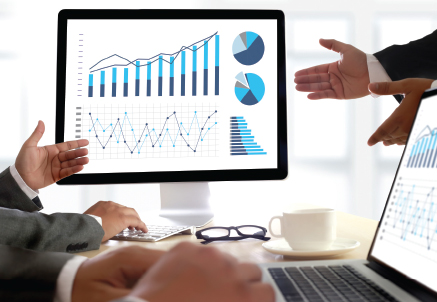
Reader Feedback
We want to hear from you! Please send us your comments and questions about this topic to InTechmagazine@isa.org.